At Simply Business, using data and analytics to make informed decisions is deeply embedded in how we work. To enable and foster this data-led culture, we have built a modern data and analytics platform tailored to our needs. This platform is shaped around three principles:
- Owning the data: Data is a highly valuable and strategic asset. Owning, managing and knowing how to leverage it is essential to our success.
- One platform: To leverage data effectively, it must be centralised in a single platform for easy access, reuse, and to enable a shared understanding. Silos, both internal and external, are the top reason why companies fail to realise value from data.
- Data & Analytics is everyone’s job: We strive to empower all departments with the data and knowledge they need in order to make decisions on their own. This is the only way to be agile and move fast.
The following diagram represents the data flows and the platform architecture:
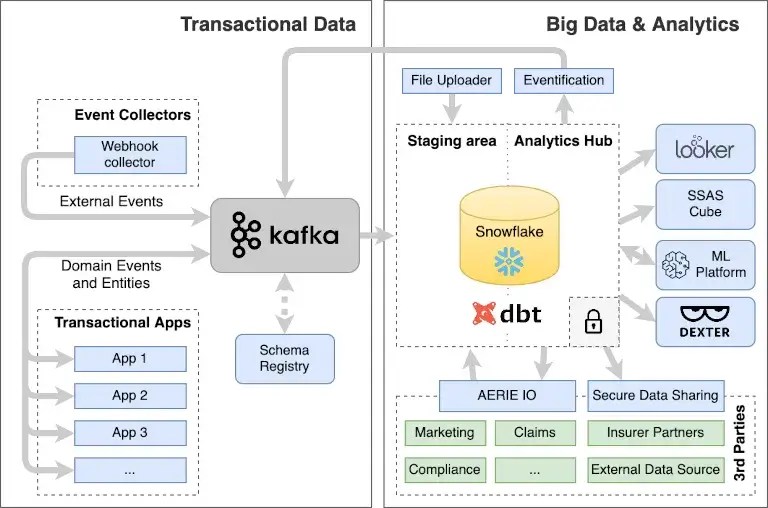
Transactional Data
On the transactional side, Kafka is our data backbone for asynchronous communication. We are slowly but surely moving from a monolithic to a distributed architecture to increase team agility. As we do it, we are getting inspiration from the data mesh paradigm to ensure that teams own the data of the services they create, while following a common approach to data governance.
A key piece for this standardisation is our schema registry, which is derived from the Snowplow open source registry and based on JSON Schema. Engineers from across the company use it on a daily basis to collaborate on data definitions.
For our main data pipelines and real-time data management tools we are increasingly using Kotlin, Kafka Streams, and Spring Boot. These technologies, in addition to improvements in our containerised CI/CD pipeline, have enabled us to increase team velocity and decrease the time needed to onboard new developers.
Eventification is an interesting project that allows us to bridge the analytics and the transactional world. We use it to check for complex conditions in our data warehouse and then fire domain events for the matching records. Those events are then consumed by transactional applications and trigger downstream processes.
Big Data & Analytics
For big data processing, we have standardised our stack around dbt, Airflow and Snowflake. They have allowed us to massively increase our development speed while strengthening our data governance practices. We are using them for all batch data processing needs: data modelling, analytics, reporting and data science.
In fact, we are building a multi-tenant analytics platform around these tools for other teams and departments. It’s hard to overemphasise the benefits of a shared toolset, which allows anyone to easily find, understand, reuse, and improve data sets.
For business intelligence and simple exploratory analysis, our main tool is Looker. All departments in the company have dashboards and Looker Explores that allow them to understand and react to what happens in their area.
We are leveraging SQL Server Analysis Services for financial, budgeting and planning matters. It provides quick access to top-level information and native Excel integration. We are looking for ways to better integrate this piece with the rest of the stack to simplify maintenance, but we haven’t found a good solution for the Excel integration and writeback support.
To ingest and safely share data with partners, we built a system that we called AERIE IO. It provides many of the standard connectors that you can expect from such a tool and it allows teams to implement custom API integrations. We are also starting to leverage Snowflake Secure Data Sharing, which avoids copying data among many other benefits.
Our ML Platform is based on AWS SageMaker but heavily customised to fit our needs. For example, it provides easy integration with Snowflake and with our containerised CI/CD pipeline. The platform allows for both notebook-based advanced analytics and production-ready ML systems.
We also built Dexter, an application to track and monitor our experiments. This is key to ensuring that we are being rigorous with our learning and that the solutions that we build have the desired impact! This was especially true in 2020, when the pandemic affected so many things we took for granted.
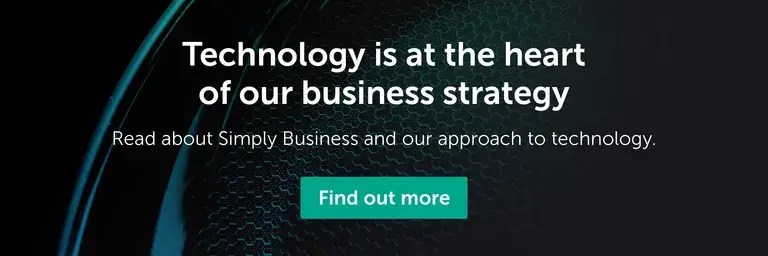
Looking Forward
Our platform allows us to innovate much faster and with much-improved data governance practices than ever before. Comparing it to how it was just a few years ago, makes you realise how much change and innovation the data and analytics space has experienced.
One of the biggest shifts we see is the progressing integration of the transactional and analytic worlds. Some of the drivers are:
- In an increasingly agile environment, systems and processes change constantly.
- Even though there’s a move to microservices, applications are becoming smarter and usually require access to more contextual information.
- In processes that have humans involved, operational analytics are becoming table stakes.
- Machine learning is blurring the lines between online and offline data processing.
With that in mind, we believe that continuing to invest in a self-serve platform that empowers teams to own their datasets is a must. For that, we choose powerful and versatile tools that can be used in different use cases. It’s not only good for reducing the cognitive load of the people working with them but also allows us to improve our data governance.
See our latest technology team opportunities
If you see a position that suits, why not apply today?
We create this content for general information purposes and it should not be taken as advice. Always take professional advice. Read our full disclaimer